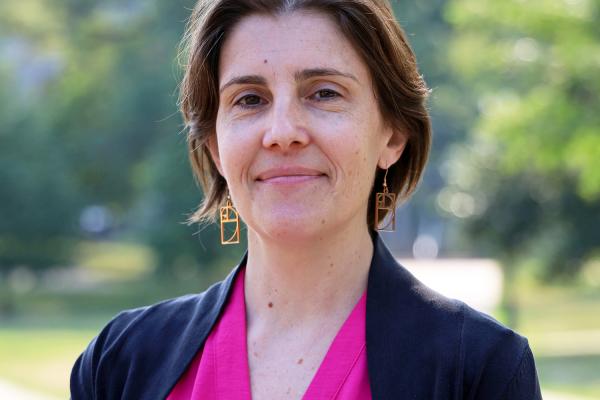
Rebecca Andridge, Professor, Biostatistics, The Ohio State University
Tile: Problematic Polls and Suspect Surveys: Assessing the Potential for Non-ignorable Selection and Nonresponse Bias
Abstract: Pre-election polls that missed the mark. COVID-19 vaccination surveys that drastically overestimated vaccine uptake. Accurately measuring public opinion and behavior is becoming more and more challenging with declining response rates and increased costs. One often overlooked possible explanation for inaccurate polling or questionable survey estimates is the possibility of non-ignorable nonresponse or sample selection – the possibility that whether or not a person participates in a poll or survey is a function of characteristics the survey is trying to measure. For example, likely voters who support a particular candidate might be less likely to answer a telephone poll about voting intention, or adults who are “anti-vaccine” might be less likely to respond to a survey about the COVID-19 pandemic and its impact on their lives. In this talk I will describe using a statistical methodology called proxy pattern-mixture models to estimate the potential impact of nonignorable nonresponse or nonignorable selection in polls and surveys. The method will be motivated by and illustrated on a set of pre-election polls in “swing” states conducted by ABC and the Washington Post in 2020 as well as two very large internet-based probability surveys (Delphi-Facebook, Census Household Pulse) that drastically overestimated COVID-19 vaccine uptake in the U.S. in early 2021. I will describe the data and assumptions necessary to implement the method and discuss challenges unique to each application. My hope is that future polls and surveys could use this methodology to provide additional bounds on published estimates, especially when sample sizes are very large and traditional confidence intervals are very small.